Table of Contents
Gesture recognition through Brain signals
Gesture recognition through Brain signals. The center of interest of this chapter is that how to differentiate the raw data signal and extract that part of the signal that would be our nature of interest or the better selection of classification. There are no significant boundaries between the boundaries of Brain Machine interface, moreover it would be difficult for a user to detect where one stage has been ended and from where other stage has been started (stage differentiation is difficult).
In many cases, preprocessor is mostly used as a classifier module. But it doesn’t happen always, at preprocessor there will be a specified transformation of raw data(signal), but the classifier hold variable which are adjusted by a guidance procedure. In order to conceptualize the working of preprocessor, there are two operations for the matter of interest, feature removal/extraction and feature chosen/selection. Feature extraction includes, collection of data (numeric digits) for the entity being categorized. Operation may include physical measurement (could be length, mass or weight) of a particular entity.
Operation may include certain complexity, it could be estimating the Fourier transform of a radio-spectrum signal in order to estimate the power in particular frequency band. Feature selection includes, the selection of required data in order to classified the particular entity. In other words, it includes selection of those feature which would be helpful in classification.
The requirement for the quantity of features being categorized should be minimum (as of complexity). There can be many reasons behind this requirement. The general potential of the classifier would be worsened if the extent(dimensionality) of input signal exceeds above than the required level. This problem is known as curse of dimensionality. In addition, large number of adaptive classifiers may cause complexity to the work as the training time is directly related to the dimensionality of data. Feature choice can also be defined as estimating a new design/pattern by the combination of two or more selected features. In this scenario, feature choice can be used as a part of feature removal/extraction. As the abstraction of both terminologies has similarity in it, so it is difficult to distinguish between them.
What is EEG Signal Preprocessing?
Signal of brain, sensed by mind-wave sensor hold data regarded as a time-changing aggregate. Brain Machine Interface, referred with extraction of corelated features from raw data signal and transforming those features, turn in to logical control in order to control home automation appliances. Signal Processing techniques have many mathematical techniques and algorithms.
• Multi-pathway processing.
• Frequency domain analysis.
• Time domain analysis.
• Spatial domain analysis.
EEG Signal Modeling
The Hodgkin and Huxley Excitation Model has discovered the generation of current signal Excitation of electrical signals from neurons in axons, replicates the structure (squid axon model) of this model. This model has same pathways as axon holds. Nowadays, Simulator for Neural Networks and Action Potentials (SNNAP) has been discovered for generation of current signal.
Non-Linearity of EEG Signal
Electro-encephalography (EEG) signal is produced when an array/beam of concentrate neuron hit the electrode of the sensor. Human brain has a complex structure have been subjected to many biological trials as it shows a nonlinear behavior. Chaos Theory and dynamic inspection proved to be useful in considering the nonlinear behavior of EEG signal. Moreover, no one till today has discovered or considered the complete complexity of human brain, even nowadays no successful model of human brain has not been provided with greater accuracy.
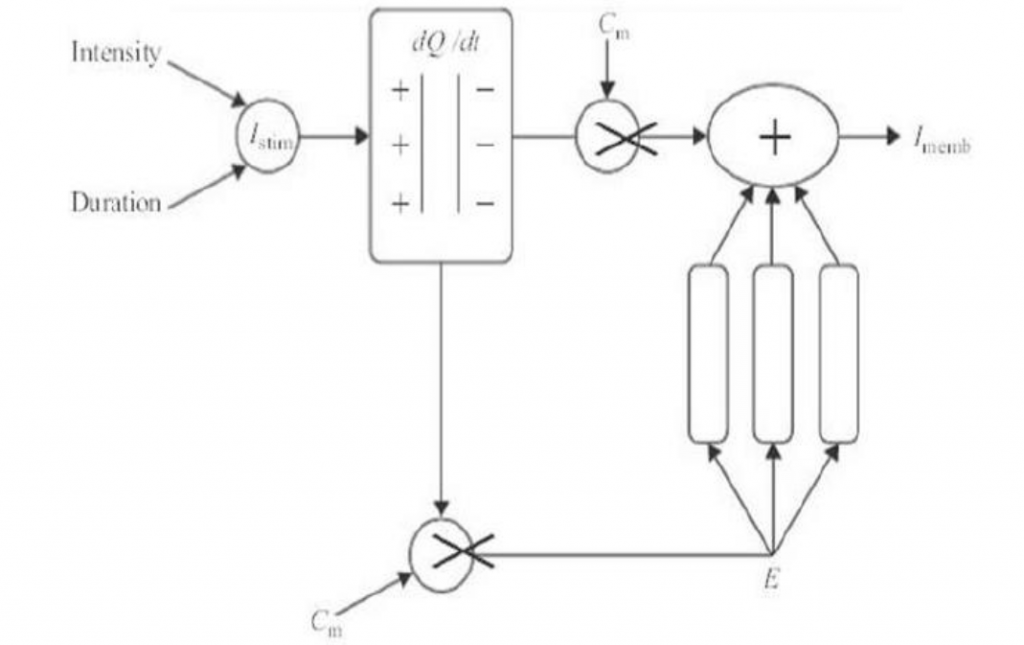
EEG Recording Methods
In order to estimate the brain signals, following technologies are used:
- Electroencephalography (EEG).
- Magnetoencephalography (MEG).
- Functional Magnetic Resonance Imaging (FMRI)
Every technology has different functionality aspect to record a brain signal. Every technology has its own advantages and flaws. Magnetoencephalography (MEG) and Functional Magnetic Resonance Imaging (FMRI) as compared to Electroencephalography (EEG) are quite expensive and are usually implemented in labs. Functional Magnetic Resonance Imaging (FMRI) has low bandwidth resolution than that of Electroencephalography (EEG). Electroencephalography (EEG) has many advantages than rest of both technologies. Firstly, it has low cost and can be easily transferable. There exist disadvantages too: Electroencephalography (EEG) has little spatial intention, which in turn required greater number of potential electrode and can cause large skull impedance(unwanted).
In past, Electroencephalography (EEG) estimation can be done using galvanometers and at a specific instant string galvanometer can be used to observe the photographic behavior of brain signal. Now a day, EEG estimation can be determined by the following stages:
- Electrodes attached with amplifier in order to abstract out the brain signal.
- These brain signal contains raw data that should be filter out.
- Lastly, it should be presented in a sting system register.
To observe Electroencephalography (EEG) estimation, data collected from brain signals is in analogous form. For Brain Computer Interface (BCI), raw data should be in digital form, analogue to digital converter should be required. EEG signals are usually estimated in 𝜇 volt that are sampled at a minimum frequency of 200 samples per second as their effective bandwidth near about 100 Hertz. At first, signal should be amplified and the accumulated to analogue to digital converter in order to have a hold on data(information) received from brain signal. In addition, to keep the effect of noise as less as possible, filter should be used before and after the operation of converter.
The characteristics of a high pass filter is to alleviate the effect of low frequency constituent (found in human breathe) and the purpose of a low pass filter is to diminish the effect of high frequency constituent (found in snoring). Purpose of notch filter is to diminish the effect of 50 Hertz frequency of electrical power supply.
EEG Electrodes.
Brain wave sensor has two electrodes:
• Dry electrode unit (Electrode that actual read the brain signal placed on the front of head).
• Reference ground connection (Ground electrode placed on the earlobe).
Electrode Positioning.
Positioning of scalp electrodes play a vital role in estimating the brain signals and furthermore to control home automation application. A standard positioning method known as 10/20 system is used world-wide, in order to estimate the position of electrodes. INTERNATIONAL FEDERATION OF SOCIETIES have originated this 10/20 system for EEG. Spot of every electrode (shown in figure 3.8) are tagged in a concealed lobe and in half of sphere position.
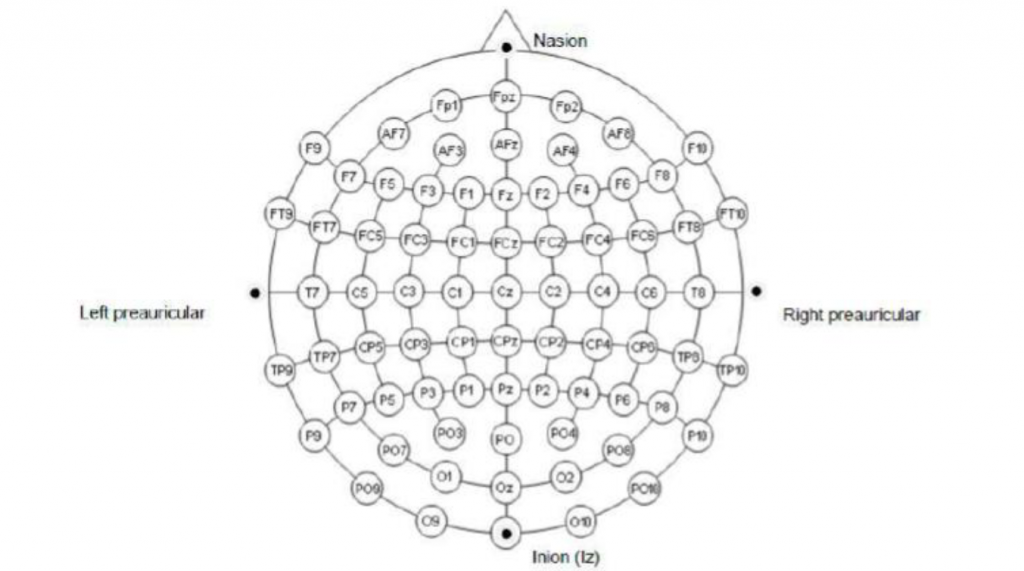
Letters are assigned according to the lobe name.
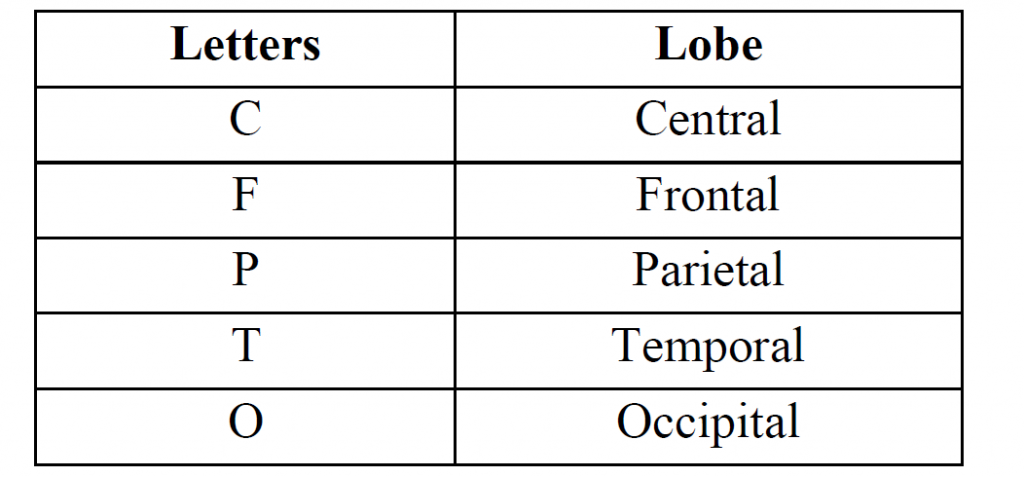
Numbers (Odd and even) are designated to particular part of sphere.
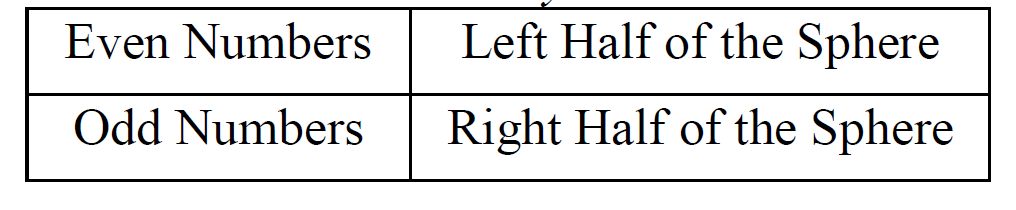
In order to determine the mechanism behind the EEG positioning, two arbitrary indicator and position of rest are determined by comparing with these arbitrary points.
EEG Considerations.
Complexity exists in estimating, analyzing and processing of an Electro-encephalography (EEG) signal. Electro-encephalography (EEG) not only provide information about brain signal but are also concerned with abnormal behavior of human’s brain. The unusual sequence is classified in three groups: 1- Extensive irregular steady wave malformation; 2- Two sided- steady Electro-encephalography; 3- Central steady Electro-encephalography. Number of neurons in a human central nervous system depends highly with the age. Aged people have very few large neurons as compared to a young one. This fact decreases the drift of blood in cerebrum.
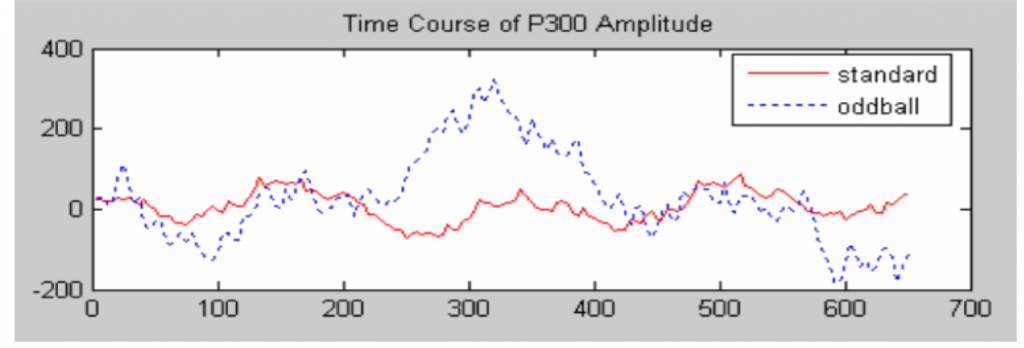
Criteria for Evaluation.
Many parameters are involved in evaluating criteria for system design in order to compare outcomes, received by sensor. As, gadget used in this project are exposed to home-based environment, may cause high dispersion for Brain-Computer-Interface (BCI) , in the form of noise. An authentic technique should be designated if device would be used by many users. The below parameters must be required for a feasible BCI implemented in home-based surrounding. It can be done by:
• Less price
• Good quality
• Better performance
• Should be easy to use and understand
• Plug and play
• Not harmful
• Electroencephalography based
These characteristics must be found in an BMI solution. Compromise of reliability would cause bad impact on user. Most important parameter is that, it should be easy to use and understand (as of complex structure). Installation and maintenance should be easy for each user. Most important is, it should be secured to operate it and must be easily transferable (from one place to another in home-based environment).
Hardware of Brain-Machine-Interface composed of: A substantial hat or several electrical wires attached with respective electrodes. This composition made a strenuous condition to implement in home-based surroundings. It would be difficult for a user to handle such complicated device.
Moreover, several numbers of wires for respective electrode, raise question about safety of user, as wires contains electricity. Electrical precautions must be considered in order to make the user safety as first priority. Second, it must be portable, as it must be used by more than one user and can easily be removable and wear on, in case user move from one place to another in home-based surrounding. Third, it must be, easy to understand and use (user- friendly). For BMI (Brain-Machine-Interface) device, it is assumed that the sensor read brain signal of user, if attenuation(noise) has a strong value, concentration of user will be distracted or deteriorate constant focus of user.
This case would be difficult for handicapped people, who aren’t even able to keep the electrode on right position, to make a strong meditation signal. Ideally it is required that the user must operate device independently, but it is not possible for those having no support to correct the position of electrodes, or a constant monitoring (24/7) wouldn’t be possible.
Moreover, intelligible and easy set of instructions should be provided to the user in order to control the BCI home-based environment device. Plug and play give a most predominant standard for a sturdy and authentic solution.
Most important issue, that the user of BCI device can face is the issue related with peripheral device or internal commands issue as user lacks technical and practical skills. Moreover, Brain computer Interface (BCI) device is totally user-dependent. Requirement of a handicapped user may vary from user to user.
Effective solutions have lift plenty of efficacious predicament for exposing the devices in the brain, long lasting potency and efficiency of embedded devices. All invasive devices (infrared gadgets) and relatively invasive device (Eastern Cooperative Oncology Group device) solutions are not related to BCI devices.
However, such solutions entail surgical intercession, but these solutions can’t voluntarily obtainable for each user and would sustain enlarge price. While there is various non-invasive technique to receive brain signals or imaging outcomes, therefore Electro-encephalography is considered as a most reliable technology. Other available technologies, Magnetoencephalography (MEG) and Functional Magnetic Resonance Imaging (FMRI) are quite costly and are not easily transferable.
Also read here