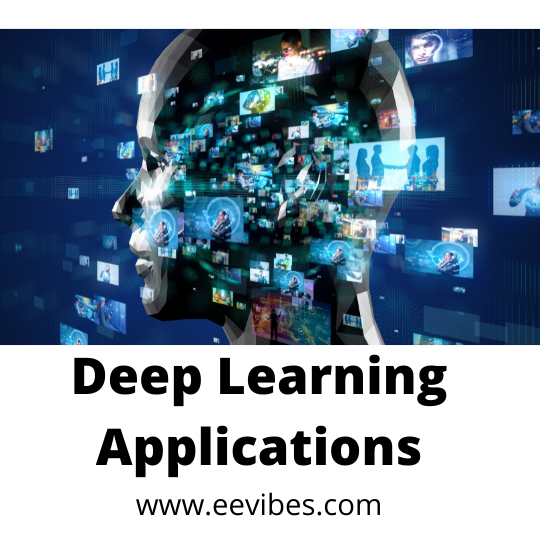
In this article I will give a short review on deep learning applications in different areas. This is the golden era of Artificial Intelligence (AI). Machine Learning is being used for most of the AI applications. While another technique that is almost kind of machine learning, is proving to be more effective and accurate is called deep learning. Thus deep learning has paved its path towards success in very short span of time.
AI has a great impact on our daily lives. Since when people have started relying on automation, machine learning has helped a lot to make it possible. From object detection to recognition phase, for classification, Natural Language Processing (NLP) , data mining to social network analysis, we can find how AI has made its place with a very fats pace.
Table of Contents
Deep Learning for Scene Understanding
The goal of obtaining a human like vision for machines is the new demand of era. Scene understanding is one of the interesting applications of deep learning where we want to train the computers for understanding and describing any image presented to them. They should also be able to decide whether it is an indoor or an outdoor image. If there is some text is present then machines should interpret how that text is related to image. Because of the limitation in traditional algorithms, the scene recognition has not progressed so well in previous years. But thanks to parallel computational hardware that have helped for training deep and complex architecture of neural networks. Scene recognition will be helpful for automatically generating captions for images.
Main Components of Scene Understanding
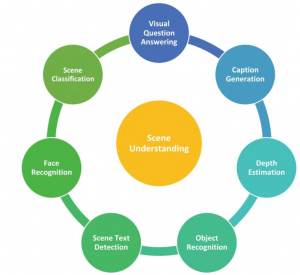
Deep Learning for Driverless Vehicle
Automation is one of the largest industries of 21st century. It aims to give more safety, reduced cost and more productivity. Many industries are working in producing the driverless transportation for future. Deep Learning is the key technology which has potential to introduce autonomous feature in vehicles. Recent studies have shown that autonomous vehicles have the potential to reduce energy up to 20%. Traffic sign detection and recognition systems are installed on the cars. These systems capture a 2D image and then through object detection and recognition different traffic signs can be recognized. Sometimes in foggy and rainy weather conditions when drivers can not pay the attentions properly, these systems can be useful for recognizing the traffic signs and help them in driving.
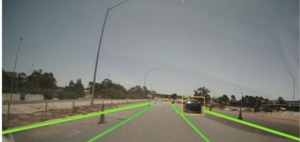
Deep Learning for Person Re-Identification in Surveillance Videos
CCTs cameras are installed in many areas and it is sometimes required to re-identify a person captured in them. Re-identification has many applications like malls, schools, colleges, offices for safety purposes. There are also some restricted areas where unauthorized person is not allowed like embassies and laboratories. Identifying a person in CCTV camera has many challenges like the lightening condition and different poses make the problem more complex. From an image or a video sequence, a human is detected as a pedestrian, then we conduct the person tracing within the camera and finally deep learning models are used for reidentification. As a result of reidentification, we obtain all similar images of the person of interest.
Deep Learning for Gait Analysis for Security and Health Purposes
We must first comprehend the techniques that our patient or athlete needs to move in order to really comprehend diseases or injuries in human locomotion. Moving from point A to point B is often thought of as occurring through gait (walking or running). Understanding precise asymmetries connected to how we move is made easier with the aid of gait analysis. Kinematic (spatial/temporal) and kinetic (force) data can be used to measure gait. A complete gait cycle, consisting of a foot strike with the observed leg, a contralateral foot strike with the other leg, and a subsequent foot strike with the observed leg, is required for a typical gait evaluation.
Human motion is a significant spatio-temporal pattern since it can serve as a strong predictor of human identity and well-being. Human gait, in example, presents a distinctive mobility pattern of an individual. The term “gait” relates to the study of human and animal locomotion. The synchronization of the brain, spinal cord, nerves, muscles, bones, and joints, as well as other body parts, is known as biometrics. Gait analysis has been researched for many different applications, including biometrics, sports, healthcare, and many more. The analysis was previously limited by the nature of the measurements obtained by the gait sensing modalities because it was mostly carried out by human observation utilizing parameters and features that were already defined in existing practice.
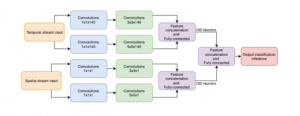
Deep Learning for Marine Species Recognition
An essential component of the efforts to conserve the ocean ecosystem is research on the identification of marine organisms. Additionally, it is a neglected application field in the world of computer vision. However, as deep learning technology has advanced, there has been a rise in interest in this subject. We provide a thorough overview of computer vision methods for identifying marine species in this chapter, primarily from the angles of detection and classification. We pay special attention to documenting the development of several deep learning methods in this field. We contrast modern deep learning methods with more established machine learning methods and talk about how the two approaches complement one another.
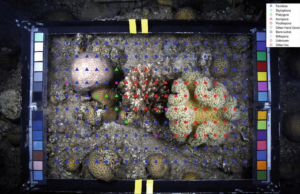
Also read here